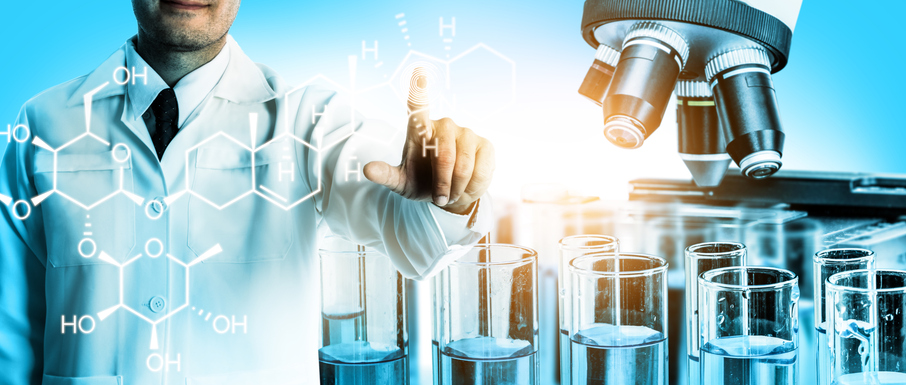
Integrating AI and Machine Learning into Clinical Trials
Integrating AI and Machine Learning into Clinical Trials
It is anticipated that in terms of AI and machine learning, 2024 will be a transformative year. AI and machine learning specialists have been attempting to incorporate effective algorithms for these fields into other sectors. Every nation's global economy is understood to be driven by the healthcare sector, and since the epidemic, public concerns about healthcare have grown.
When discussing the healthcare industry in particular, several subfields must be handled effectively. Ensuring efficient and seamless healthcare operations is crucial, encompassing drug discovery, medical imaging, and other clinical trials. To incorporate AI and machine learning into healthcare, scientists and developers have worked together, which could have a good impact on the healthcare sector.
Transforming Clinical Trials with AI and Machine Learning:
Artificial Intelligence and Machine Learning have significant potential for improving research operations, clinical trial designs, and general management results. Algorithms can identify areas for protocol optimization, such as appropriate endpoint selection, sample sizes, and study lengths, using data from previous clinical trials.
More effective and instructive research is the result, which lowers expenses, accelerates timelines, and eventually raises the likelihood of positive results. Moreover, the ability to decipher complex data enables researchers to plan experiments that lessen participant attrition while raising patient burden.
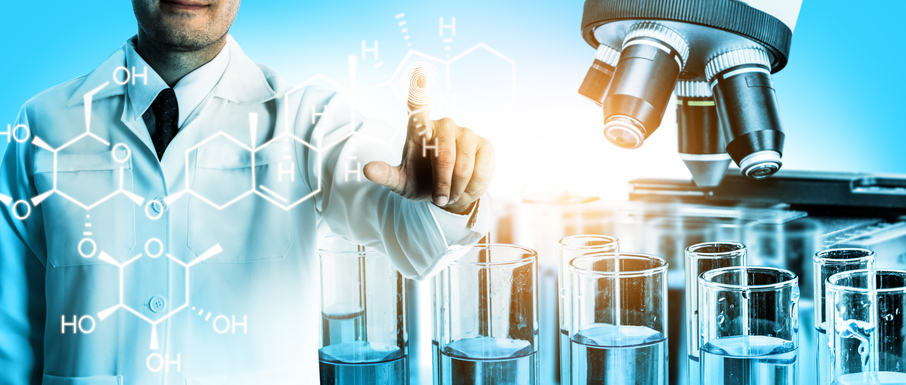
How do AI and Machine Learning help in Clinical Trials?
Study Design:
When creating databases and case report forms (CRFs), automating the build stage can be quite important. A lot of CRFs were manually generated by data managers and administrators in the past, which was time-consuming and occasionally led to human error. In 2024, artificial intelligence and machine learning have found their way into the healthcare sector.
The question, "How does AI reduce human error?" comes to mind. AI reduces the possibility of human mistakes in several ways, but in the healthcare industry, its integration with machine learning has improved the study design stage of clinical trials and increased the efficiency with which results are produced.
Patient Recruitment and Management:
The most time-consuming aspect of a research endeavor is typically finding patients. Data managers have to find and categorize a set of subjects, gather information, and use inclusion/exclusion criteria to narrow down the field and choose participants. Effective patient recruitment is essential to the success of a trial.
To increase productivity, quality, and retention in patient management and recruitment, artificial intelligence (AI) and machine learning algorithms can be used. These algorithms can gather subject data while qualifying and sifting possible participants. They can use data sources like medical records and social media information to identify subgroups and regions that may be relevant to the experiment. AI can also inform medical personnel and patients about clinical trial prospects.
Electronic Health Records (EHRs):
Experts in AI and machine learning contend that improving patient data management can be greatly aided by incorporating these technologies into clinical trials. Since the answer to what is natural language processing has become well-known, scientists have found ways to integrate NLP into healthcare.
Healthcare experts claim that with the aid of AI and machine learning, matching patient data and extracting pertinent information from an unstructured EHR becomes simple and less time-consuming. Because natural language processing (NLP) is involved, the process also becomes more effective and guarantees accuracy in the final product.
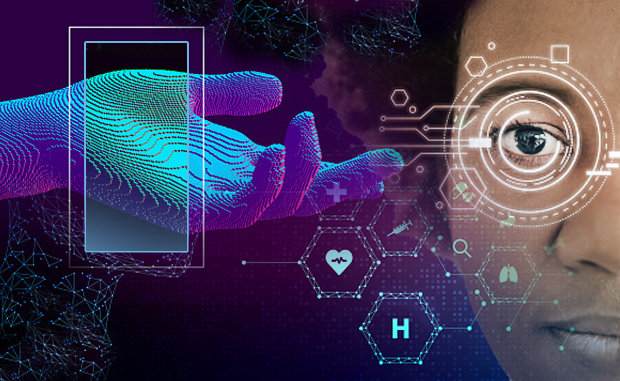
Real-Life Application of AI and Machine Learning in Healthcare:
Feasibility Assessment:
The procedures and approaches utilized for feasibility studies a few years ago were labor-intensive, resource-intensive, and prone to human error. By putting these algorithms into practice in 2024, clinical research operations have improved accuracy, expedited data gathering and management, and taken less time.
Patient Enrolment and Recruitment Processes:
A critical stage in each clinical research is patient recruiting and enrollment. To keep track of each patient's medical history and other health-related data, effective patient data management is essential. The management of Electronic Health Records (EHRs) is improved, data collecting is streamlined, and the amount of time required to complete the process is decreased by integrating AI and machine learning algorithms.
Clinical Research:
Healthcare research and studies take a lot of time and can be very costly. It costs money to access research articles and to experiment with methods and medications using different tools and gear. Such equipment can occasionally experience failures, and repairs are expensive. Buying many large-scale pieces of equipment is less expensive when AI and machine learning are used in clinical research, and the results of investigations and research are better.
Final Thoughts:
The application of AI and machine learning to clinical research is causing a major upheaval in the pharmaceutical industry. The fact that this technology was adopted so quickly and the large number of studies that have been published in the pharmaceutical sector demonstrate how crucial these technologies will be in changing clinical research in the future.
By leveraging AI and machine learning, pharmaceutical companies may accelerate drug discovery, enhance patient outcomes, simplify clinical trials, and usher in a new era of innovative healthcare. Clinical research's incorporation of rapidly developing technology portends an infinite future for the field, driving it toward ground-breaking discoveries and a more effective, patient-centered approach to clinical trials and drug development.